Let’s face it. Recommendations are hard. The main reason is that recommendations entail implicit communication. And communication is a human skill, related to language and its meaning.
Going back to the time we went to a video rental store. The more you went, the more at ease you felt talking to the video clerk. And the more you spoke, the better she knew you.
You shared tastes, mood, and other details. The information you shared and your pre-watched movies gave the video clerk a pretty good idea of the next film to recommend to you.
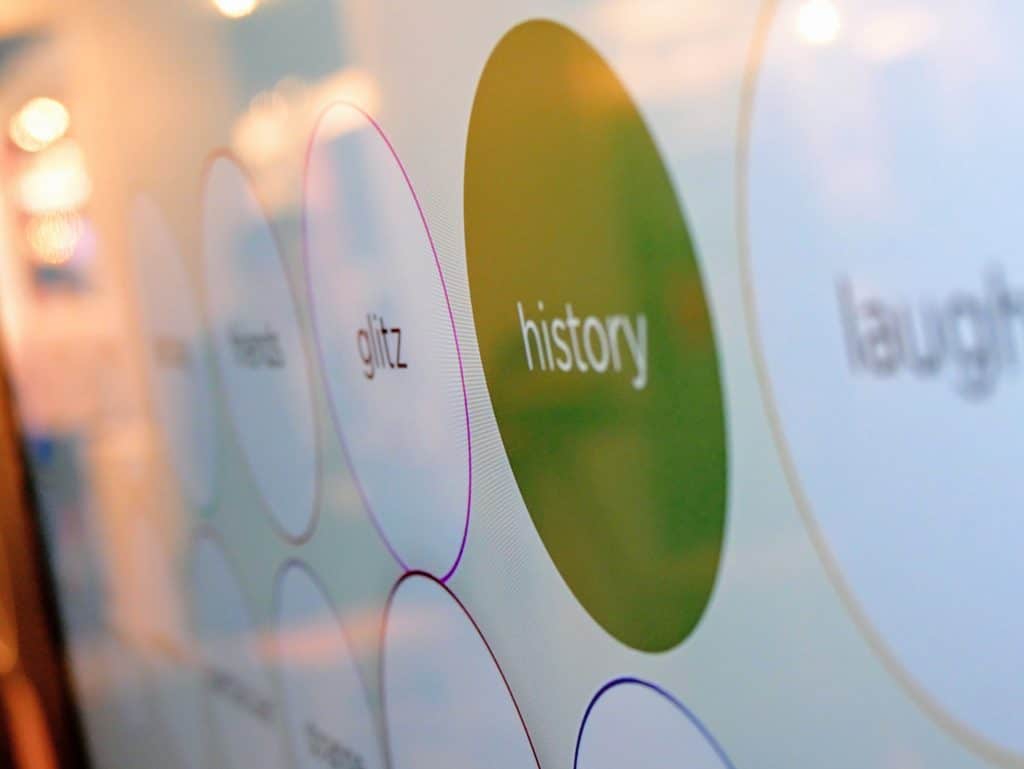
We all want this personal experience when we subscribe to a video-on-demand (SVOD) or television service. We want to be at the video rental store. We want the relationship we had with the video clerk.
The secret behind the formula for humanized recommendations is communication. And behind great communication, you have language and semantics.
So that is why Spideo offers a content recommendation and analytics platform that uses semantic-based discovery. It delivers personalized viewing suggestions based on natural language, profile, and social trends.
Improving recommendations using semantics
Spideo recognized the value of semantics from the beginning.
I am a movie lover. So they use the semantics related to my past interactions to recommend the next film.
What does that mean? They create a semantic fingerprint.
As in the video rental store, a semantic fingerprint combines data and people.
- ‘Data’ is all the movies I ever watched.
- ‘People’ is the video clerk herself, and the empathic conversation she keeps with me, which allows her to understand my context. Through our conversation, she knows how I am feeling and what I care about, based on other movies I’ve watched.
The people behind humanized recommendations
Data history, by itself, brings no novelty to recommendation systems. Many of these systems work based on what I watched already. Also, they work based on what others have watched as well.
The value of your data gains meaning when you can:
- associate meaningful words to my interests;
- name content titles and show them in my preferences;
- explain why you believe a specific content is relevant for me; and
- empower me to act on the data you have on me, and learn from the consequences of my behavior.
So Spideo works with data scientists that develop algorithms aiming to find the right data points to explain the reasoning behind recommendations. However, Spideo also works with data crafters that add feelings to data, using common words and natural language to classify and match content with my preferences.

We can help to improve recommendations
Remember the video rental store? If we’d choose not to interact with the video clerk, she would never be able to recommend the next movie.
As viewers, you and I are strategic pieces for better recommendation systems.
It’s a two-sided conversation.
Data crafters make Spideo speak naturally to us.
We interact with Spideo to improve recommendations.
Moreover, unlike other recommendation systems, Spideo empowers us with control by providing all the tools and endpoints.
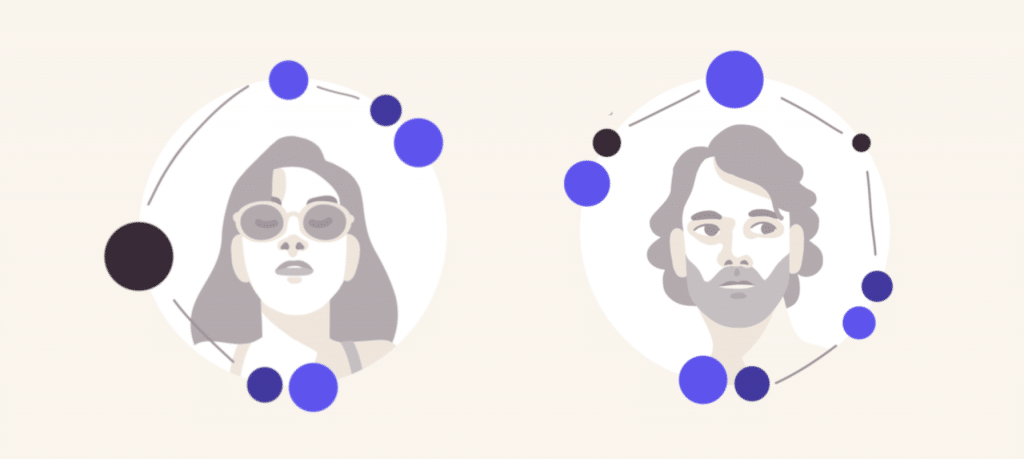
So, we have the power to decide what and how much information to provide. And then Spideo uses the history of our interaction and associates intelligence to increase the value of the recommendations it makes.
Recommendations sound sweeter already
A semantic fingerprint is when Spideo puts relevant words on both content’s characteristics, and our tastes and interests.
The semantic fingerprint is the formula for recommendation success. It combines data semantics and user semantics, putting people behind each step:
- data scientists, who understand consuming patterns;
- data crafters, who classify content based on natural language; and
- all of us who love to watch your content, who interact with your system and provide critical information for future recommendations.
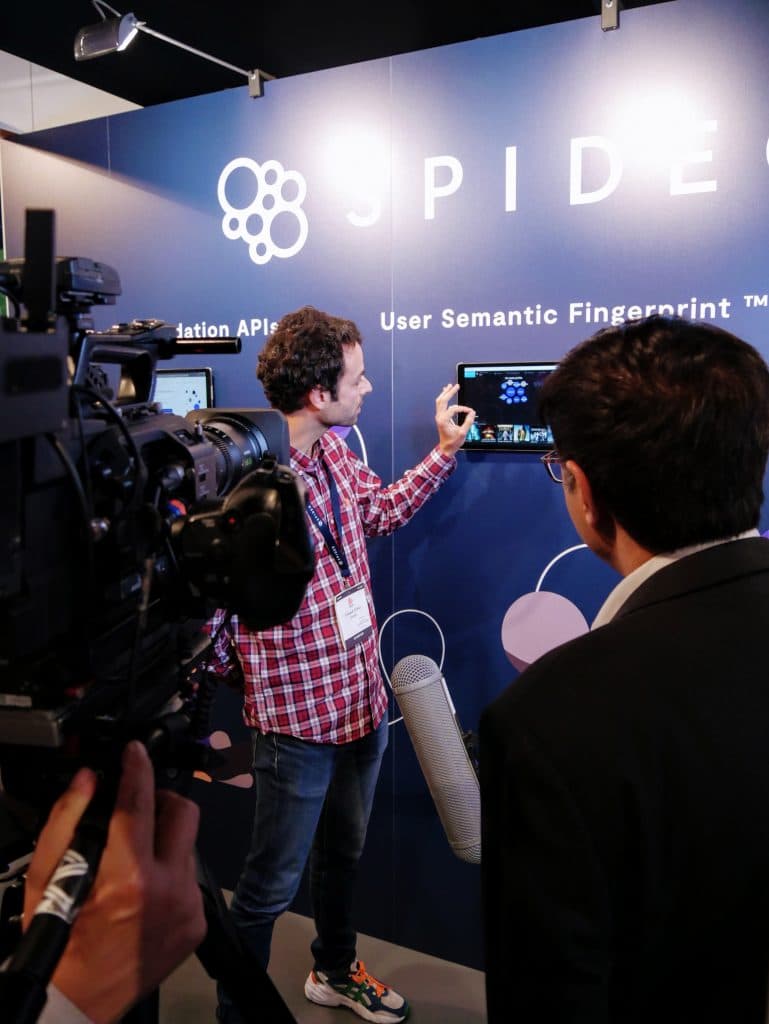
So without this, you’re only able to relate basic demographics and straightforward information about consumption habits. You have information about what I watched, but nothing on my motivations.
Semantically enhanced recommendations bring a deeper understanding of motivations, preferences, and moods of users.
Do you still believe recommendations are hard? Turn your recommendation system into the video clerk next door.
Further reading
“Smart Data,” Spideo
“Privacy by Design,” Wikipedia
“De la Privacy by Design à la Privacy by Using,” Réseaux 2015/1 (n°189), pages 15 à 46, Alain Rallet, Fabrice Rochelandet, Célia Zolynski
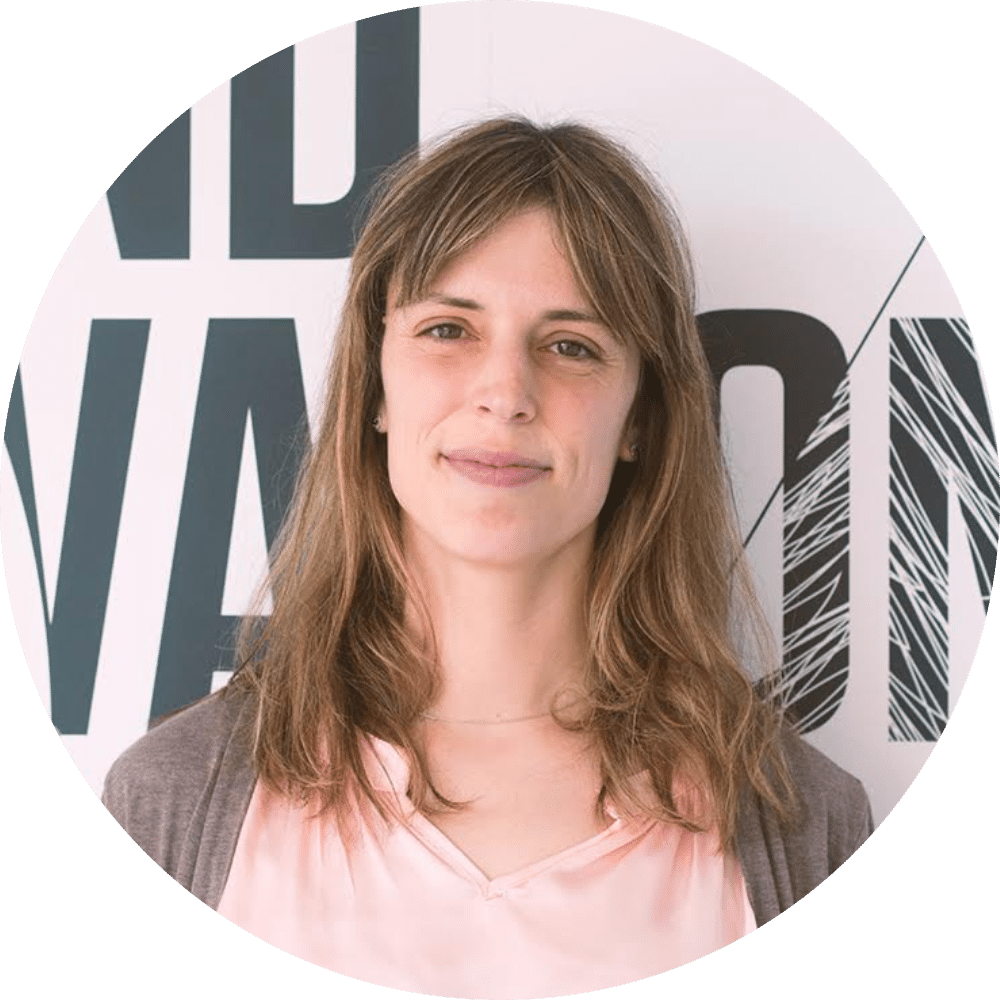
WRITTEN BY